Enterprise Fraud Management Solutions Types: Pros & Cons
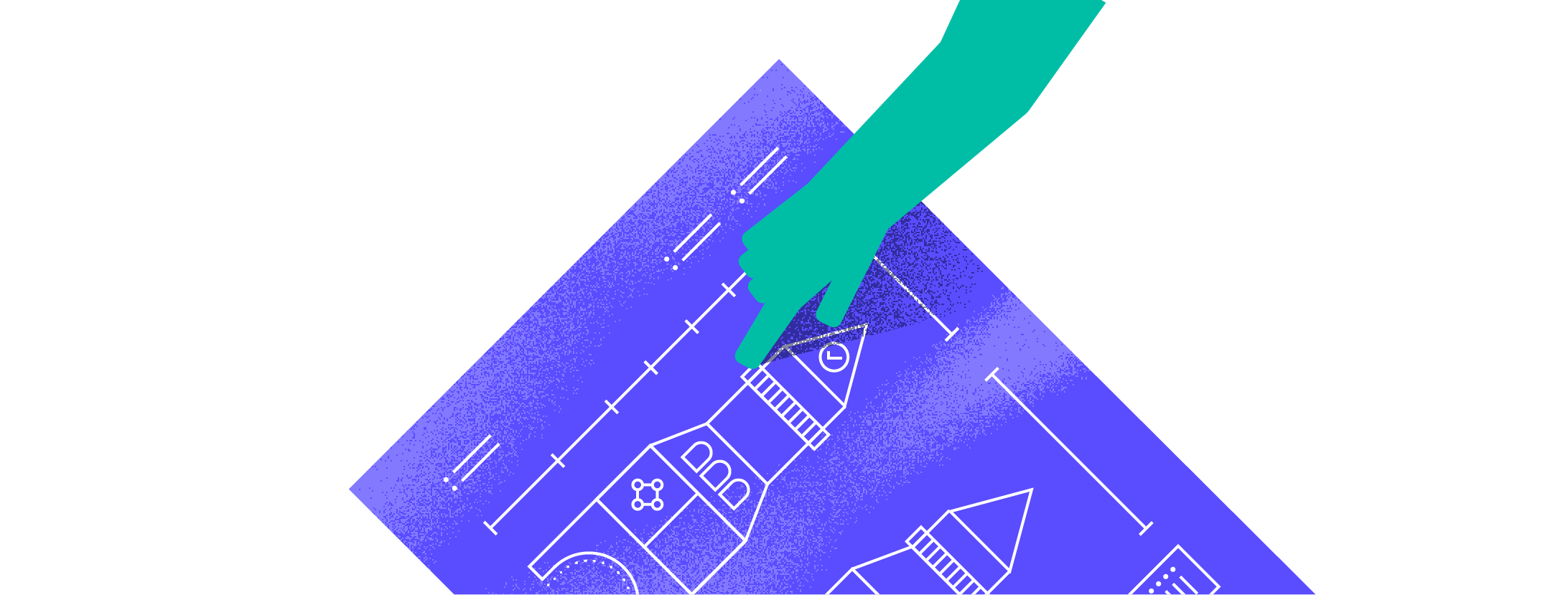
This is the third blog post of a five-part series.
Here is part one: The Fraud Prevention Space’s Complex Optimization Problem
Here is part two: What You Can Gain by Partnering With a Fraud Prevention Vendor
If you are already searching for a new fraud prevention solution you’re probably looking to improve your performance. You’ve likely defined the goals you’d like to achieve by partnering with a new vendor. The purpose of this blog post is twofold:
- To help you understand what types of solutions are available
- To list the pros and cons of each one
But first, an important note: Partnerships between merchants and fraud prevention vendors last 3-5 years on average. And once you integrate with a vendor, only a catastrophe will make you switch. For this reason, it is critical to ensure the right decision is reached.
The world of fraud has evolved in the past 5-10 years. eCommerce is ever-changing, fraudsters are becoming much more sophisticated and machine learning technology has become the dominant new tech innovation. Before you can choose a fraud prevention solution, you should know and understand the different types of solutions, technologies and business models available.
Rule-based solutions
Before machine learning became the hot topic of the tech world, fraud prevention was conducted by creating a set of rules to prevent fraudsters from placing orders. These sets of rules need to be tweaked and updated regularly since fraud patterns evolve constantly. Whether it’s entering a new market or selling new products from new categories (for example, expanding from accommodation to flights, or adding digital gift cards) the fraud patterns change.
Machine learning solutions
The great advantage of machine learning solutions is the ability to categorize and analyze thousands of data points in a much more coherent and efficient way than the human brain. Machine learning solutions are much more proactive and are designed to learn from their own mistakes so they can improve their performance and accuracy even without any human intervention. Two key factors affect accuracy and model performance:
1) The data that is being used to train the model.
2) The quality of the data science work in creating the relevant features, industry, and market expertise.
Scoring solutions
When sending a transaction to a scoring solution, the service will not send back a decision but rather a recommendation in the form of a score. The merchant will then need to decide what to do with the score – approve, decline, send to 3DS, or check manually. In this case, the merchant is the one who stays liable for the chargebacks. Usually, the business model for scoring is charging a fixed fee per transaction, whether they were eventually approved or declined.
Chargeback guarantee solution
Unlike scoring solutions, chargeback guarantee solutions provide a decision and not a recommendation. The decision is binary — either to approve or decline the transaction. If the decision was to approve the transaction and it eventually returned as a chargeback, there is a shift in liability, and the fraud prevention provider will reimburse the merchant for the fraud-related chargebacks. Usually, a chargeback guarantee solution will charge a percentage fee, only for approved transactions, and the fee will be set based on the risk profile of the transactions.
Let’s take a look at the pros and cons of each type of solutions:
Rule-based
Pros:
- The merchant has full control over the rules that are being created and used.
- The merchant can relatively easily apply their own inputs and translate them into rules to prevent fraud.
Cons:
- Rules are reactive and not proactive. Rules don’t learn by themselves and are slow to react to changes in fraud patterns. Hence, they allow fraud to come in, until rules are tweaked to fit the new patterns.
- Managing and tweaking the rules requires a significant amount of resources. Many times, merchants will not find the time to do it often enough and therefore they leave fraudsters with an easy way in.
- Merchants may often reach a point where they have hundreds of different rules without the ability to know how a new rule actually affects their performance.
- Maintaining a rules system while expanding to new markets, payment methods and / or products might be very risky, since the existing rules might not be effective for the new markets or products. Expanding to new markets means that the merchant doesn’t have the expertise and knowledge to create a new set of rules, which means that by the time he will gain this knowledge, he is exposed to high fraud risk.
- The merchant is exposed only to its own data only and therefore, cannot proactively predict fraud patterns and adjust accordingly. The merchant will adjust to new fraud patterns only once the merchant is exposed to new fraud behavior, which might already be too late.
Scoring
Pros:
- Scoring solutions give merchants full control and discretion regarding whether to approve, decline or manually review transactions.
- Scoring tends to be cheaper than chargeback guarantee on a per-transaction basis.
Cons:
- Scoring solutions have an inherent incentive misalignment with the merchant. If the fraud prevention solution is not performing very well, the merchant is the one suffering from it by having high chargeback rate or sub-optimal approval rate, while there are no real consequences on the fraud prevention provider that gets revenue per processed transaction.
- Scoring solutions almost always require a manual review team. Since the scoring solution provides only a recommendation, most merchants will use a manual review team to review ‘grey area’ transactions. A manual review team is not scalable and can affect customer experience, often leading to suboptimal performance (for further elaboration see, What You Can Gain by Partnering with a Fraud Prevention Vendor.
- It is hard to determine what the right threshold (score) is to determine whether to approve or decline an order. For example, if you decide that every transaction that receives a score lower than 12 will be declined, what do you do with a transaction with 12.14 score? The threshold constantly needs to be adjusted and some transactions will inevitably fall close to the set threshold. If you decide to have a bit more of a complex fraud operation, you might decide to include 3DS for ‘ambiguous’ scored transactions. With this option, a merchant is risking that this segment will encounter high consumer drop-off rates and high decline rates that are typically associated with 3DS.
Machine learning
Pros:
- Allows for hundreds or thousands of decision trees to be aggregated into one model to make a more accurate decision.
- Proactive technology that knows to learn from its own mistakes and improve even without human intervention.
- A proactive solution that uses a wide network effect, allowing the model to identify and mitigate fraud patterns and fraud rings early on, and automatically adjust.
Cons:
- It is very complex to create good and accurate machine learning models. Models need to be industry-specific and sometimes geo-specific. Some vendors take pride in using machine learning technology when in reality their performance is sub-optimal and machine learning is being used as a buzzword to attract merchants.
- Some vendors expect merchants to take an active part in building or improving the machine learning models using the merchant’s data science team. This might be very complex and not within the merchant core capabilities, require maintenance (like maintaining rules), not really effective and lead to suboptimal performance.
Chargeback guarantee
Pros:
- With chargeback guarantee solutions, the incentives of both vendors and merchants are aligned. They charge only for approved transactions (that generate revenue for merchants) so they strive to approve as many orders as possible. They are also liable for fraud-related chargebacks, which means they seek to keep chargeback rates low.
- Chargeback guarantee solutions provide a definite approve or decline decision for each order. This simplifies the merchant’s fraud operation as there is no need to adjust fraud thresholds or have additional verification layers.
- Chargeback guarantee solutions ensure a strong and constant focus on R&D which is the essence of any AI solution. Since the vendor is responsible for the chargeback cost, it is their imperative to mitigate the latest and most innovative fraud attacks promptly while maintaining a high approval rate. Therefore, they put a strong focus on R&D to develop tools to proactively and accurately uncover fraud patterns, creating an inevitable R&D value loop.
Cons:
- Chargeback guarantee solutions usually have a minimum monthly cost which makes them more suitable for larger enterprise merchants than to SMBs with low monthly online sales volume. Chargeback guarantee solutions charge a % fee from each approved transaction, so the merchant needs to make sure that the ROI from using a chargeback guarantee solution is positive.
Equipped with a better understanding of the pros and cons of the different types of solutions, we are ready to dive into the deep water. In the next blog post, we will cover the main considerations for choosing the right fraud prevention partner for you and what questions you should ask during the evaluation process.