The ABCs of Machine Learning and How It Boosts Fraud Management
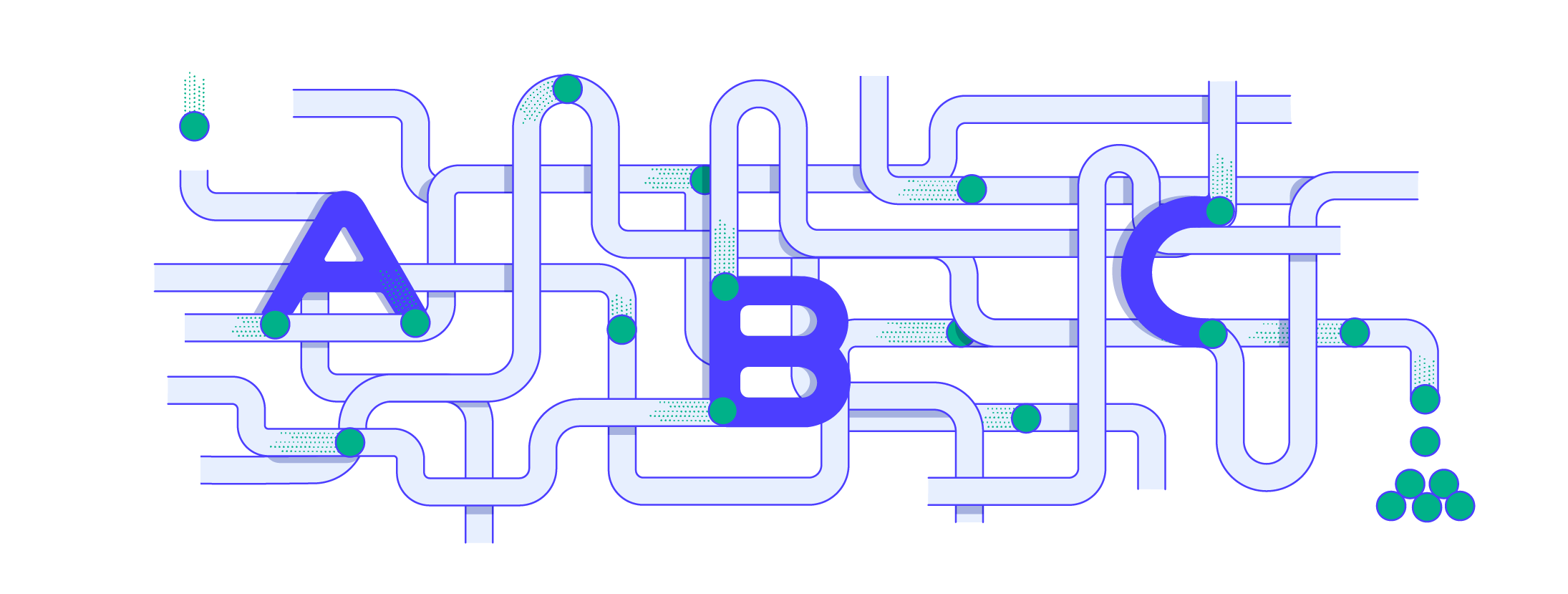
eCommerce has seen innovation like never before.
Consumer demand is driving merchants to expand globally and offer new and innovative omni-channel flows. And as business is changing, fraud is getting more and more complex. But even in this ever-changing landscape, many merchants are still using outdated rule-based systems that are struggling to keep up. And even worse – they’re forcing merchants to expend resources to constantly tweak and adjust them.
As opposed to timely rules, machine learning makes fraud management scalable. By leveraging this technology, merchants can continue to innovate, not just in the realm of fraud management, but also toward a better customer experience, which can translate into revenue growth through customer life time value.
In this post, I’ll explain why machine learning outperforms traditional rule-based fraud prevention systems.
Adaptive Decisioning
Millennials are always on-the-go, in fact, Riskified’s consumer survey found that 45% of them use their smartphones to shop online. Nevertheless, as recently as two years ago, over 90% of merchants were still using a single strategy to analyze both web and mobile transactions. So, a rule designed to review desktop orders would not take into account geographical data that is unique to mobile transactions. Let’s say a US college student traveling in Europe was trying to book tickets from their mobile device for an upcoming concert in New York, using their American credit card: from a rules perspective, this would appear as an IP/BIN mismatch and the a decline would be more likely.
How can machine learning help?
Machine learning models, do not use set rules. Instead, risk factors and their relevance are determined based on the most current patterns, as reflected in the huge amounts of realtime order data. The models evaluate thousands of data points, including IP and device, to refine decisioning based on the most influential data combinations of an order at any given time. The result is a more accurate and adaptive understanding of shopping patterns.
Better Data (with the Help of Features)
Riskified’s research revealed that nearly one-third of shoppers who were wrongly declined abandoned their purchase entirely or went to a competitor. This doesn’t just mean a missed sale for the merchant, but more importantly, wasted time and lost loyalty of a valued customer. An effective way to counter this is to let go of stringent rules. Rules are essentially black and white, and such rigidity simply cannot account for the hundreds of variables involved in identifying fraud. The reason models are able to evaluate a transaction with such accuracy and speed is a result of features, which are a critical part of machine models.
Features are the data elements used in a machine learning prediction task– they can be simple like order amount, or more complex like a match between keyboard language and the credit card’s country of origin. The value or weight of a feature correlates to its contribution to the overall level of risk. This is at the core of how models help contextualize data.
What does this mean for fraud?
What really stands out about features is that they can be dynamic. Dynamic features allow models to react in real-time as fraud develops. A dynamic feature might be a specifically risky IP and BIN combination, for example, an Indian IP with a French credit card. If there is a sudden peak in fraud with this IP and BIN combination, the risk level would be immediately adjusted across all models with this feature. The effect of this is faster, more accurate predictions and fewer false declines. For the merchant, this means models are able to continually detect risk across emerging fraud schemes.
Contextual Training
Future-proofing is always something important to keep in mind when thinking about a fraud management solution. Rules are not easy to time-proof. They require significant in-house resources to stay relevant. Machine learning models, on the other hand, are able to improve accuracy at scale.
How do models do this?
Through a training process, the model is refined using the most accurate representation of the current fraud landscape. A good way to think about this is to look at the holiday season. Spikes in international orders and expedited shipping are common as shoppers are rushing to get the best deals and their gifts delivered in time for friends and family. These spikes would jump out as highly risky for a model trained pre-holiday and the accuracy would be compromised. That’s why training is not one-size-fits-all. Rather, each model has a unique way of measuring success depending on how, where and when the model is applied.
By magnifying unique shopping behaviors, models make more accurate predictions on unseen data, no matter the season. For a merchant, this means a malleable fraud management solution that gets better with time.
The bottom line
Machine learning can help merchants manage the complexity of today’s eCommerce landscape. To learn more about our models and technology stack read about how we use elastic linking as part of our review process.